Adapting programs in a time of rapid change
COVID-19 is a time of flux. In a matter of months, we’ve seen social and economic upheaval across the globe.
In times of uncertainty and transition, change-making organisations need to be agile and responsive. Our survival and our ability to help others depends on it. With a few tweaks, a well-embedded measurement, evaluation and learning (MEL) system can be a vital resource for adapting programs in a time of rapid change.
Theory of change and learning loops
As practitioners, we are well aware of the benefits of MEL for adapting programs, albeit in more stable periods.
Theory of change processes help organisations work out what to do, what to measure, and how to set up the systems for collecting and interpreting data. Most programs use two “loops” of learning to make decisions, to use a concept popularised by Chris Argis in the early 90s.
The first is “single loop” learning, which is related to implementation. This is usually utilised by program staff closest to the action who will frequently reflect on what’s working and what’s not.
The second is “double loop” learning, which is related to broader strategy. This learning process is usually done by the decision-makers at the helm of an organisation, who need to reflect on whether the goals and activities are still relevant to the context and whether their big assumptions still hold true. These double loop decisions happen less often, maybe every few months or twice a year.
When the context is constantly shifting, there is an even greater need to take a learning and adaptive planning approach. In the context of COVID-19, we’ve identified two key changes to make. The first is to shorten the cycles of data collection and feedback. The second is to ramp up your double-loop learning.
Let’s unpack how you’d change your approach to learning and adaptive planning by walking through an example we often use to teach theory of change at Clear Horizon Academy: the carpark model.
An example of adaptive planning: the carpark model
Imagine that you’re the owner of a carpark in the CBD. Before the pandemic, your main challenge was that cars were being stolen and this was discouraging parkers. Your vision for your carpark was that it would be safe and profitable, free from car thieves and full of happy customers.
You had created a theory of change to transform your carpark, and through this process decided that adding CCTV cameras was the one major influencing activity that would increase the number of customers.
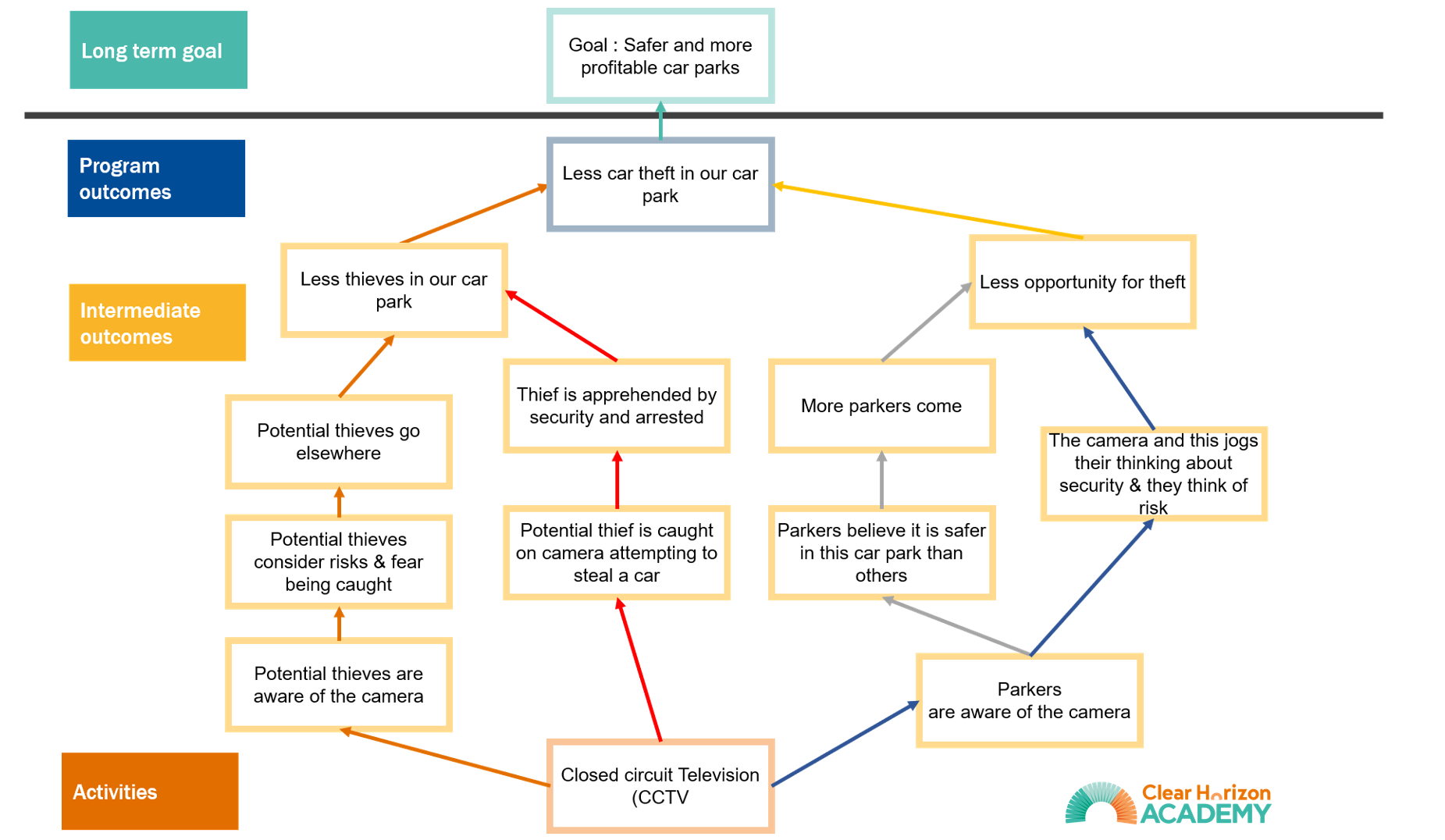
The carpark model’s theory of change.
For a while, this had worked. Not only had the CCTV cameras deterred thieves, but they had also attracted more parkers. This had created more eyes in the carpark, creating ‘natural surveillance’ and reducing opportunity for theft. Times were good, and your change program was working.
Then, COVID-19 hit.
Now with everyone working from home, there are a lot less people driving into the city. There’s a hospital nearby, so a few workers are still parking here. But each day there are less customers, and theft is starting to spike.
You realise that COVID-19 has created major changes in your context that are inhibiting your goals. So you decide to shorten your cycles of data collection and feedback, with a focus on double loop learning.
You take the following steps:
Step 1
The first aim is to gather data about this new context, with more regularity. You set up systems for single loop learning: daily reports from the carpark attendants on their observations, as well as pulse surveys for customers to understand what is concerning them. You set up a weekly time to review CCTV footage to try to spot patterns in the thefts and understand their cause.
Step 2
Armed with information, now is the time to use double loop learning to revisit the theory of change. Are your broader goals still relevant? Do we still need a safe and profitable car park in this context?
You determine your goals are still relevant. However, you note there is a need to broaden the definition of safety. From your weekly scans, you realised your customers are concerned about hygiene in the carpark – so you redefine safety in terms of security as well as health.
Step 3
Now you’re moving toward adjusted goals, you need to reconsider your activities. Will they work and be effective in this new context? What might hinder or help each causal pathway to your goals?
You realise that one of your major assumptions is no longer holding up. In a pre-COVID-19 world, you assumed that more people in the carpark would create natural surveillance, discouraging thieves and attracting more customers.
But from your data scans, you find out that a bustling carpark is having the opposite effect! Customers are concerned about being close to others and having more people touching the ticket machines, which increases their chance of catching COVID-19.
Step 4
You come up with additional strategies to work with your revised assumptions. To balance between natural surveillance and social distancing, you prohibit parking in every second car space. You also install contactless payment technology and put up signs highlighting your public health-consciousness. As you begin to implement, you use your new single-loop feedback systems to check whether these strategies are working.
What we can learn from this example
For any organisation trying to continue their important change work, this approach can greatly help us with adapting programs in a time of rapid change like COVID-19. Regularly gathering implementation data and scheduling time to re-assess your strategy will set you up to do your best in this chaotic period.